In the first game of a five-game series of Go between human and machine, hardware beat out wetware, with Google DeepMind forcing an international champion, Lee Sedol, to forfeit. Which means that Google DeepMind is one step closer to that sweet sweet $1 million dollar winner’s prize (which is great, ’cause Google is so strapped for cash), as well as the title of top Go player in the world.
Though DeepMindâa company that was bought by Google in 2014âhas been developing their “AlphaGo” program for only a few years now, it has already beat one of the best living players in the world: South Korea’s Sedol.
But this win is not like Deep Blue beating Kasparov in 1997, nor is it like Watson taking the Jeopardy! title in 2011. AlphaGo, as well as all of the other work being done at DeepMind, is all about AGI, or artificial general intelligence. In other words, Deep Blue and Watson were programmed specifically to play their respective games; AlphaGo on the other hand, learned how to play Go… like a human. It could theoretically be retrained to play other games just as well.
If you’re unfamiliar with Go, it is essentially a Chess of the East that was created over 3,000 years ago. However, there is one very important distinction between Go and Chess: it is, according to DeepMind CEO and co-founder Demis Hassabis, “the pinnacle of board games… [and is] the most complex game⦠ever devised by man thatâs played professionally.” There are over 10^170 possible board configurations, as well as 10^700 different possible games. Which is, if you’re keeping score, more than the number of atoms in the universe.
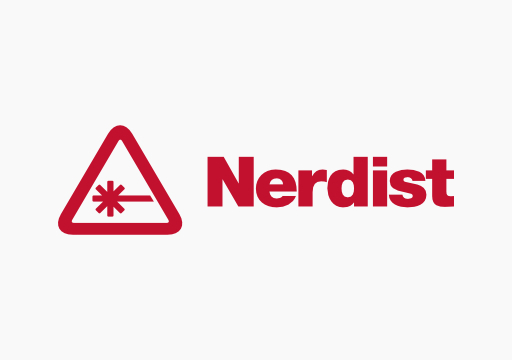
Image of a Go board. There are only two rules in Go, and the goal is to capture as much territory on the board as possible.
This huge number of possible game configurations, as well as options for any given move (there’s an average of 20 possible moves for any given turn in Chess, and 10 times that for any given turn of Go) is precisely what made Go so much harder to crack than Chess. In fact, many AI experts thought it was going to take at least another 10 years to figure out solid AI for Go, if ever.
Using deep neural networks, however, the team at Google DeepMind was able to have AlphaGo learn to play Go by processing and playing millions and millions and millions of games to determine what moves it should play in any given situation in order to win. This is a critical distinction between a program like Deep Blue, because AlphaGo, unlike Deep Blue, couldn’t “brute force” its way to the right move (there’s simply too many possible options), so it had to essentially develop an intuition for what makes for a good move based on the data set it was fed (those millions of games).
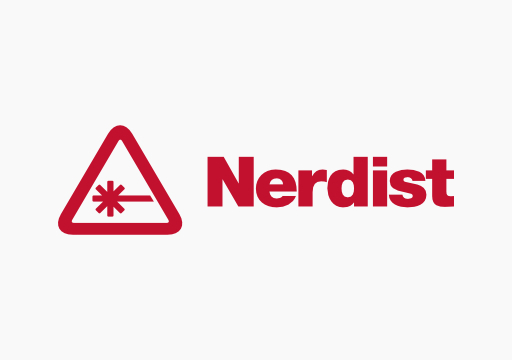
A simplified diagram of a neural network, which takes raw inputs, processes them, and turns them into actionable outputs.
If you’re wondering about applications for Google DeepMind’s AGI, the possibilities are endless. In fact, DeepMind’s mission statement has essentially always been about two things according to Hassabis: first, solving the problem of intelligence, then solving everything else. This means that the goal for DeepMind is to develop a general artificial intelligence that’s so smart, it will be able to solve humanity’s problems, whether they be in climate science, search functionality, or even high-energy physics.
While this may seem like some seriously Cyberdyne-in-the-sky kind of thinking, keep in mind that DeepMind has access to Google’s seemingly endless coffers and computer power, as well as Alphabet’s (the company that owns Google) other enterprises like Boston Dynamics.
There are still four games to go in the series, however (which can be watched live here on March 10, 12, 13, and 15), so we’ll have to wait and see if AlphaGo can claim another one of humanity’s titles (and seemingly human-only endeavors) for the machines. But make no mistake, DeepMind is looking to make computers at least as smart as we are. As far as Hassabis can tell, “there doesnât seem to be anything non-computable in the brain.”
Let’s hope that’s a good thing…
What do you think about AlphaGo beating one of the best Go players in the world? And where do you think AGI is headed over the next few years? Let us know in the comments section below!
—
Images: Google DeepMind, fdecomite